Multimodal AI Foundation Models in Earth Observation Unlock Power of Remote Sensing Data
Apr 29, 2024
In a recent editorial published in the Innovation Geoscience, researchers from the Aerospace Information Research Institute (AIR) with the Chinese Academy of Sciences (CAS) highlight the significant advances in multimodal AI foundation models in Earth observation techniques, particularly in unlocking the power of remote sensing big data, and their profound impact on our understanding of the planet's various facets.
Remote sensing, which involves gathering data from space without direct contact, has become instrumental in providing insights into areas such as land and air quality, as well as the well-being of ecosystems and living organisms.
One of the key challenges faced in utilizing remote sensing data is the sheer abundance of information from diverse sources like satellites and airplanes. Current methods often struggle to effectively process this vast amount of data and extract meaningful insights. To address this challenge, researchers have turned to artificial intelligence (AI) techniques to develop smarter systems capable of interpreting remote sensing data more efficiently.
A notable advancement highlighted in the editorial is the emergence of multimodal AI foundation models. These models integrate various data types, including images, text, and videos, to enhance our understanding and analysis of Earth's surface and environment. By unifying different data modalities, these models represent a transformative shift in optimizing remote sensing big data for a wide range of Earth observation objectives.
The article also discusses challenges such as the fragmentation among existing techniques and the need for consistent solutions for handling diverse types of remote sensing data. Researchers propose establishing a unified multimodal remote sensing foundation model to streamline data processing and improve efficiency in Earth observation tasks.
Furthermore, advancements in pretraining techniques, particularly in spectral remote sensing data, have led to the development of models like Spectral GPT. Trained on extensive datasets, these models have shown promise in tasks such as scene classification and change detection, demonstrating the potential of AI in Earth observation.
In conclusion, the editorial underscores the significance of multimodal AI foundation models in revolutionizing Earth observation. By harnessing the richness of remote sensing big data, these models offer a robust framework for addressing complex Earth observation applications, paving the way for a transformative era in our understanding of the planet.
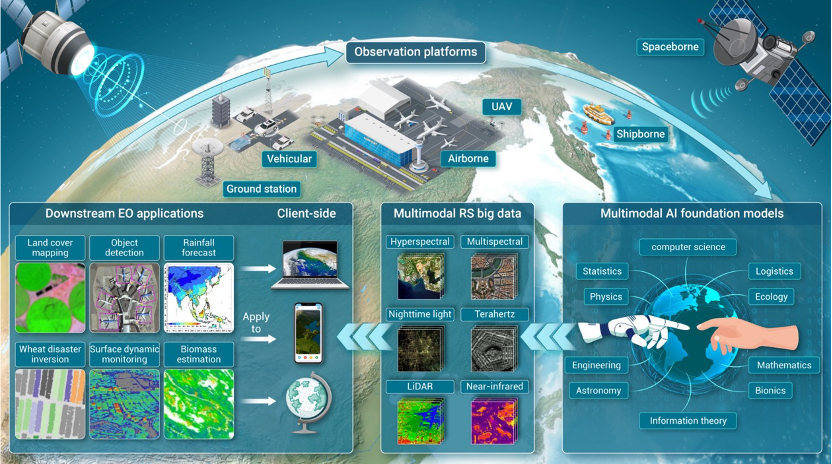
A cycle-chain RS intelligent interpretation system enabled by multimodal AI foundation models for RS big data in EO.
News & Events